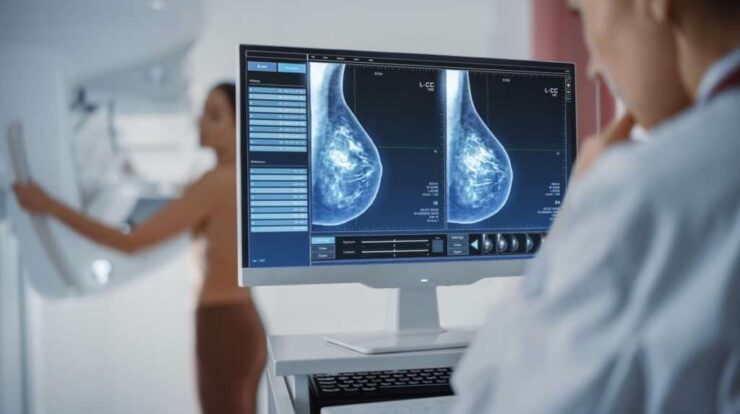
Between planned mammogram appointments, certain breast cancers might quietly develop and advance. Such instances are referred to as intervals.
breast cancers
, or Interval Breast Cancers (IBCs). Unlike cancers detected through routine screenings, interval cancers typically come to light when a patient starts showing symptoms prior to their subsequent mammography appointment. Because these cancers often prove more aggressive and challenging to address, timely detection becomes crucial.
A recently published study from the
UCLA Health Jonsson Comprehensive Cancer Center
indicates that artificial intelligence might aid in identifying these cancers earlier—potentially even before they can be seen with the naked eye. Thanks to AI, scientists think it’s feasible to decrease the instances of overlooked cases and enhance patient results.
AI Provides a Secondary Review of Overlooked Indicators
Dr. Tiffany Yu, an assistant professor of radiology at UCLA and the primary researcher behind the study, highlights why inflammatory breast cancers pose such difficulties: “Identifying these interval cancer cases sooner allows for detection when the disease is more manageable. Early diagnosis significantly benefits patients as it often leads to milder treatments and enhances the likelihood of achieving favorable outcomes.”
Intervals cancers may occur between annual screenings in the U.S., where individuals usually receive digital breast tomosynthesis, commonly referred to as 3D mammography. Conversely, many European nations depend primarily on standard digital mammograms.
mammography
, or 2D screening, and recommend screening every two or three years. The differences in timing and technology can affect how and when these cancers are caught.
unlike numerous European initiatives, American screening programs typically refrain from categorizing interval cancers as part of their routine procedures. This lack of classification makes it challenging to evaluate the effectiveness of these screening techniques or explore potential assistance from technologies such as artificial intelligence. To tackle this issue, the UCLA research project was designed with the objective to bridge this gap.
A Look at the Data
The research team examined approximately 185,000 mammography scans between 2010 and 2019. Among them, they found 148 instances of interval cancers.
breast cancer
. Using a classification system borrowed from European guidelines, radiologists then categorized the cancers based on how they appeared—or didn’t—on earlier scans.
Related Stories
・
Researchers create light-activated ‘smart’ bomb to destroy breast cancer
・
Pioneering breast cancer vaccine halts tumors before they begin
・
Lifechanging discovery reduces breast cancer recurrence
These categories included:
・
Missed Reading Error:
The cancer was apparent but ignored.
・
Minimal Indicators (Executable and Non-Executable):
The cancer exhibited slight indications that might have been identified or were probably too nuanced to observe.
・
Occult:
The tumor did not show up on the mammogram but could potentially be identified with alternative imaging techniques.
・
True Interval Cancer:
The cancer was actually not there at the time of the
last screening
but developed quickly afterward.
・
Technical Error:
The scan had inherent issues.
Researches subsequently utilized an artificial intelligence tool known as
Transpara
, to these earlier mammograms. The AI scored each scan on a scale of 1 to 10 for cancer risk. Anything above an 8 was flagged as suspicious.
The outcomes were impressive. The AI system identified 76% of the mammography scans that were initially interpreted as normal but were subsequently associated with interval cancers. This encompasses:
・
90%
Of the instances where reading errors occurred.
・
89%
of the minimal-signs-actionable cancers.
・
72%
of the minimal-signs-non-actionable cancers.
・
69%
of the occult cancers.
・
50%
Of the actual interval cancers.
To put it differently, the AI frequently detected things that the radiologist missed—particularly when the indicators were not obvious.
Where the AI Struggles
Nevertheless, the tool had its constraints. Dr. Hannah Milch, who was the lead author of the study and affiliated with UCLA, noted that although the AI identified numerous cases,
occult cancers
It found it difficult to precisely determine the location of the cancer.
For instance, even though the AI tool couldn’t detect them on mammograms, it identified 69% of the screenings that included hidden cancers,” she explained. “But upon examining the particular sections of the images where the AI indicated potential issues, it performed less effectively, marking the true cancer sites just 22% of the time.
This inconsistency indicates that although the AI tool might be useful for signaling possible dangers to physicians, it should not serve as an independent decision-making source. Rather, it could function like another pair of eyes to assist.
radiologists
concentrate their focus, particularly in instances where irregularities might be easily overlooked.
Dr. Yu concurs. She stated, “Although AI is not flawless and should not be utilized independently, these results suggest that AI might assist in moving interval breast cancers predominantly into the category of true interval cancers.” This change would result in detecting more cancers at an early stage and reducing the number that remain unnoticed until symptoms appear.
The Bigger Picture
Interval breast cancers
They are not uncommon. The percentage can vary based on the program and technology employed, ranging from about 10% to as high as 38% of all cancers identified in screened groups. Some studies indicate that the incidence of IBC varies between 7 and almost 50 cases per 10,000 screenings.
The term “false negative” mammogram adds another layer of complexity—this refers to an examination that initially appears normal yet later reveals evident indications of cancer upon review. Various studies have differing approaches when it comes to classifying these: some categorize false negatives under Inflammatory Breast Cancer (IBC), whereas others keep them distinct. Consequently, the stated rates for false negatives can fluctuate dramatically, ranging anywhere from 4% to 40%.
Contributing to the complexity is the significant variation in the operation of screening programs. Elements such as the duration between screenings differ considerably.
imaging technology
The number of readers involved and even the level of radiologist training can affect outcomes. This underscores the importance of establishing standards for reviewing and classifying interval cancers.
UCLA’s study stands out as one of the initial ones in the U.S. to employ a rigorous categorization approach for extensive screenings and assess AI performance using actual case scenarios. Unlike much prior research which originates primarily from European setups, this endeavor illustrates potential applications of AI within an American setting characterized by yearly examinations.
3D imaging
are more common.
Next Steps for AI in Breast Cancer Screening
The results provide an encouraging look at what AI may potentially offer both radiologists and patients. When utilized together with skilled practitioners, AI technologies can assist in identifying minor indicators of cancer, thereby giving physicians an opportunity to intervene before tumors become more difficult to manage. However, additional studies must be conducted to improve the application of these tools and address significant concerns regarding their precision and dependability within medical environments for interval breast cancer detection using AI.
There remains much uncertainty,” stated Dr. Milch. “We require more extensive prospective studies to grasp how radiologists would actually utilize AI in their work and manage instances where AI highlights potentially problematic areas not discernible to human eyes.
human eye
.”
The research contributors from UCLA encompass Dr. Anne Hoyt, Dr. Melissa Joines, Dr. Cheryce Fischer, Dr. Nazanin Yaghmai, Dr. James Chalfant, Dr. Lucy Chow, Dr. Shabnam Mortazavi, Christopher Sears, Dr. James Sayre, Dr. Joann Elmore, and Dr. William Hsu. This investigation received backing from the National Institutes of Health, the National Cancer Institute, the Agency for Healthcare Research and Quality, along with Early Diagnostics Inc.
With the advent of more tools like this, the objective becomes evident: reducing overlooked cases of cancer, initiating treatment at earlier stages, and improving patient outcomes.
It seems like you might have started with an instruction but didn’t include the actual content to be paraphrased. Could you please provide the specific text for me to work on?
The Cheerier Aspect of Journalism
.
Enjoy heartwarming tales? Check this out
The Cheerful Aspect of Current Events’ Newsletter
.